Toyota, Hitachi, and others profit from a weak yen and rising overseas demand.
In the quarter from April to June this year, 70% of surveyed Japanese companies' net profits exceeded market expectations, with companies in the automotive and artificial intelligence sectors performing the best, as many of these businesses benefited from the favorable impact of the yen's depreciation.
Japanese media compiled the performance of about 130 listed companies that announced net profits during the period from April to June, with at least three securities companies forecasting the profits of these companies. The list excludes financial institutions and companies that do not have internal forecasts for the fiscal year ending in March 2025. We compared the average analyst forecasts from QUICK Consensus with the performance of these companies.
Toyota Motor Corporation had the largest gap between market forecasts and performance, with net profits for the quarter increasing by 2% year-on-year to 1.33 trillion yen (approximately 8.98 billion U.S. dollars), while the market had previously predicted a 7% decrease in the company's net profits for the quarter.
The average exchange rate for the quarter was about 156 yen to 1 U.S. dollar, depreciating by about 20 yen compared to the same period last year. The weak yen brought Toyota a profit of 370 billion yen, offsetting the impact of a certification scandal that led to a suspension in shipments of some models.
Advertisement
The depreciation of the yen has benefited businesses that rely on overseas demand. Honda Motor's net profit exceeded market expectations by nearly 60 billion yen. The automaker gained 47.5 billion yen in profit due to exchange rates, and its high-priced hybrid vehicles also performed well.
Suzuki Motor also received a boost of 37.5 billion yen from the soft currency, while domestic price increases offset the impact of rising labor and research and development costs.Companies related to artificial intelligence have also performed prominently, with surging demand. Hitachi's net profit totaled 175.3 billion yen, a year-on-year increase of 150%, exceeding market expectations by 70%. Its power transmission and distribution business has grown against the backdrop of active investment in data centers, and its information technology department has also performed well.
Tokyo Electron has benefited from strong shipments of semiconductor manufacturing equipment, with net profit rising by 96% year-on-year to 126.1 billion yen, exceeding market expectations by 30%.
Companies that failed to meet market expectations have been severely impacted by intensified competition in the United States and a recession in China's economy.
Nissan Motor's net profit fell by 73% to 28.5 billion yen, more than 70 billion yen lower than market expectations. The increased sales incentives implemented by Nissan Motor to meet the demand in the United States have proven to be a heavy burden.
Steel manufacturer JFE Holdings' profit margins have shrunk due to the deterioration of market conditions caused by a decline in steel demand in China.
Among the approximately 1,080 companies listed on the Tokyo Stock Exchange Prime Market, the fiscal year ends in March, and 60% of the companies' profits from April to June have increased year-on-year, nearly 10 percentage points higher than the same period last year. This list does not include some subsidiaries whose parent companies are also listed.
Japanese AI chip startup, challenging NVIDIA
Currently, Asian startups are striving to prove that they will provide better solutions than the AI chip giant NVIDIA, as the current GPU design is relatively bulky and quite energy-consuming, which will become the breakthrough for startups.
AI chips are mainly divided into "inference" and "training", the former is to make AI models generate predictions or conclusions, and the latter is the process of developing new AI models to make accurate inferences. For example, OpenAI needs to train chips to develop new models of the chatbot ChatGPT, but to make ChatGPT answer questions, inference chips are needed.
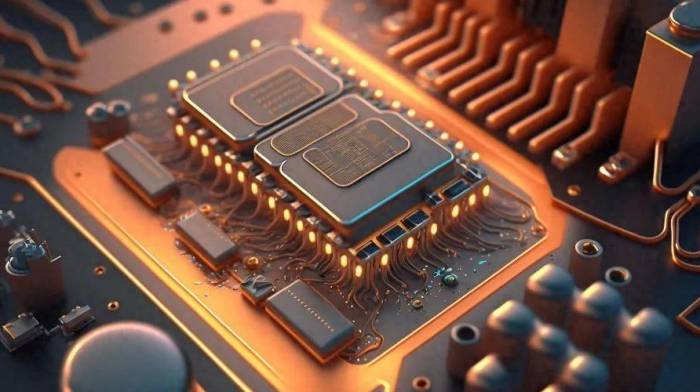
Toru Nishikawa, CEO of the Japanese AI unicorn Preferred Networks (PFN), said in an interview, "No one has come up with the perfect inference chip architecture. I think there will be a big change in the architecture of this field, and whoever provides the right architecture at the lowest price will win." PFN is developing training chips while also viewing the inference chip market as an opportunity to test the product's energy efficiency.The current success of NVIDIA is primarily attributed to the booming "training" industry. Its GPUs are capable of handling the massive parallel computations required for the development of AI models. However, due to the high cost and large size of GPUs, they are not suitable for integration into laptops and wearable devices.
The AI chips used by NVIDIA in data centers are priced between $25,000 and $40,000 each, which is 7 to 8 times more expensive than traditional products. Nishikawa believes that if the cost of inference chips is the same as that of training chips, this business model would be difficult to sustain, and it is necessary to develop AI chips that can operate on PCs.
Kazuhiro Sugiyama, Director of Consulting at Omdia, expects that the demand for "on-device AI" will increase in the future. The potential market includes PCs, smartphones, surveillance cameras, and drones, all of which encourage startups to join the competition. The primary issue with NVIDIA's products is the price; a single NVIDIA chip costs $25,000, which is a burden for companies looking to invest in AI.
Startups currently have the opportunity to challenge NVIDIA, including SambaNova Systems, a U.S. company supported by the SoftBank Vision Fund; Tenstorrent, founded by former Intel engineers; and Graphcore, a British company recently acquired by SoftBank. In addition, large technology companies such as Google, Microsoft, Meta, and Amazon have also joined the fray, as well as NVIDIA's competitor AMD.
Starting from the software to make AI chips more efficient, PFN and its partners aim to develop the next generation of AI accelerator chips by March 2027. Junichiro Makino, the head of chip design at PFN and a professor at Kobe University, pointed out that the goal is to make the new chips more powerful and energy-efficient than the B200 chipset. The company plans to start from the software because the software is similar to the "brain" inside the chip, determining how to effectively handle specific tasks and distribute data and computations to each core, allowing each core to focus more on processing task-related data.
Moreover, some startups believe that NVIDIA, in order to improve chip performance, adopts the method of increasing cores and memory, which is like "using brute force to solve problems." This is similar to a car wanting to accelerate and choosing to make the engine larger, which in turn sacrifices efficiency.
Overall, NVIDIA is also manufacturing AI chips tailored to specific needs, but the main strategy is still to adjust the software platform to meet the needs of specific applications such as AI or self-driving cars, without making significant changes to the overall architectural strategy. Sugiyama from Omdia bluntly stated, "NVIDIA is unlikely to change the architecture to something completely different from the GPU. They will always manufacture the same type of chips, which is also the reason for their economic benefits."
Industry insiders have revealed that NVIDIA also adopts a more versatile design approach, prioritizing chips that can run multiple top AI applications. Startups can focus their design on specific applications to find new competitive spaces.
Memory is also one of the breakthroughs.Japanese AI chip startup Edgecortix is attempting to address the "memory wall" issue. Although GPUs are adept at computing large sets of matrices, they lack long-term memory, hence they access memory chips during computation, recording results or recalling the next task.
The more times it has to enter and return from memory, the higher the power consumption and delay, which comes at a high cost, also causing the chip to consume additional power to compensate, leading to a bulky design and low energy efficiency. As a result, the chips designed by Edgecortix can handle data in a more streamlined manner, reducing the number of times the chip needs to access memory. When processing and computing surpass general GPUs, the company does not need powerful and expensive HBM chips.
Edgecortix CEO Sakyasingha Dasgupta pointed out that Japan's "chip revival" has also changed the environment to the company's advantage. TSMC's establishment of a factory in Japan will help cultivate the local chip design ecosystem. "From a geopolitical perspective, the Asia-Pacific region is currently in a very critical position, which is one of the advantages," said Nishikawa of PFN, who believes that Japan's chip packaging solutions are competitive.
*Declaration: This article is the original creation of the author. The content of the article is the author's personal opinion. Our reposting is only for sharing and discussion, and does not represent our approval or agreement. If there are any objections, please contact the backstage.
Comments