On July 30th, Nvidia's stock price fell by 7%, marking the largest drop in the company's value in three months, with a market capitalization loss of $193.4 billion overnight, sliding to $2.55 trillion.
From July 10th to 30th, Nvidia's stock price plummeted by 23%, dropping from $134.91 per share to $103.73. Previously, the company's unrelenting upward momentum seemed to make investors overlook the risks.
In the past two weeks, investors have withdrawn funds from large technology stocks like Nvidia, as they become increasingly concerned that large corporations' AI spending may not yield returns.
Technical analysts point out that such a shift leaves room for further decline in Nvidia's stock price.
01
Apple's Fault?
The significant drop in Nvidia's stock price may be related to Apple Inc.
On July 29th, Apple stated in a technical paper that two models of its artificial intelligence (AI) system, Apple Intelligence, were trained on cloud chips designed by Google. Apple provided detailed information about the tensor processing units (TPUs) used for training. Additionally, Apple released a preview version of Apple Intelligence for some devices.
Advertisement
In its 47-page paper, Apple did not mention Google or Nvidia, but pointed out that its Apple Foundation Model (AFM) and AFM servers were trained on cloud TPU clusters. The paper stated that the system enabled Apple to effectively and scalably train AFM models, including AFM-on-device, AFM-server, and larger models.Apple stated that AFM-on-device was trained on a single slice with 2048 TPU v5p chips, which are the most advanced TPUs launched in December 2023. AFM-server was trained on 8192 TPU v4 chips configured to work together as 8 slices through a data center network.
Google has been deploying TPUs in large quantities within its data centers early on, to accelerate AI model training and inference. Moreover, not only does Google use them internally, but it also offers TPUs as a cloud computing service to third parties, turning them into a product for sale.
The latest Google TPU costs less than 2 USD per hour, and the chips need to be pre-ordered 3 years in advance for use. Google first launched TPUs for internal workloads in 2015 and made them available to the public in 2017. Now, they are the most mature customized chips designed for artificial intelligence.
However, Google remains one of NVIDIA's top customers and sells the use of NVIDIA technology on its cloud platform.
Apple previously stated that inference, which involves using a pre-trained AI model and running it to generate content or make predictions, will be partially completed on chips in Apple's own data center.
Technical documents released by Apple during the June 2024 WWDC show that engineers, in addition to using Apple's own processors and other hardware in Apple Intelligence, along with their own software frameworks, also use their own GPUs combined with Google TPUs for accelerated training of AI models.
The competitive pressure on NVIDIA is increasing, with Google, for example, continuously expanding its market share through self-developed AI chips. Data from TechInsights shows that in the 2023 data center accelerator market, Google TPU shipments reached 2 million units, slightly behind NVIDIA's 3.8 million units, but has firmly taken the third place in the industry, with a strong growth momentum, posing a challenge to NVIDIA. At the same time, tech giants like Microsoft are also gradually reducing their dependence on NVIDIA and turning to other competitive brand chips.
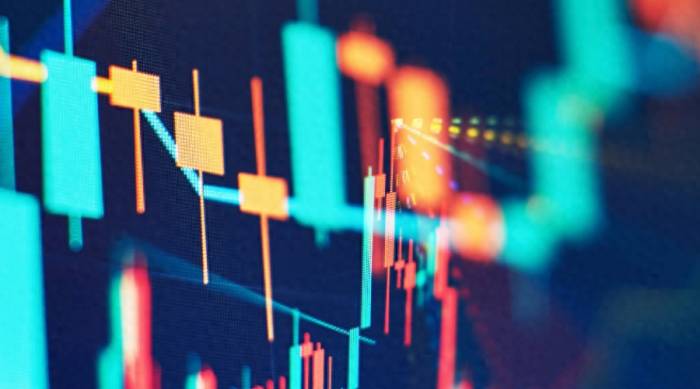
02
GPUs are too expensive
In addition to the risk of single dependence, the high price of NVIDIA GPUs has also scared away many manufacturers.Reports indicate that AI servers equipped with Nvidia's next-generation Blackwell GPU are priced as high as 2 to 3 million US dollars per unit.
Nvidia has launched two reference designs based on the Blackwell architecture. Among them, the NVL36 is equipped with 36 B200 GPU acceleration cards and is expected to be priced at 2 million US dollars, up from the previously anticipated 1.8 million US dollars. The NVL72, which is twice the scale, is equipped with 72 B200 acceleration cards and is expected to start at a price of 3 million US dollars.
Nvidia anticipates that by 2025, shipments of B200 servers could reach 60,000 to 70,000 units, with a total value of 120 to 210 billion US dollars.
Currently, AWS, Dell, Google, Meta, Microsoft, and others are interested in purchasing B200 servers, with the scale exceeding expectations.
AI servers are primarily composed of processors such as CPUs, GPUs, and FPGAs, which are used to handle a large number of computational tasks. Compared to traditional servers, AI servers typically require higher-performance hardware to meet the demand for large-scale data processing and complex computations. Due to the high cost of these hardware components, they account for the largest proportion of the AI server's cost, with GPUs being the most expensive among the various processors.
During the AI training and inference processes, GPUs are usually the most expensive hardware. This is because GPUs have strong computational capabilities and parallel processing capabilities, which can accelerate the training and inference processes of AI models. Most AI servers are equipped with multiple GPUs to meet the demands of high-performance computing.
Due to their powerful computational capabilities, GPUs also have higher power consumption. To meet the computational needs of AI models, multiple GPUs are usually configured, which further increases the power consumption of the server. High power consumption means that the server requires a larger power supply during operation and results in higher electricity costs.
Compared to CPUs, GPUs have a more complex architecture and more components, which means that GPU maintenance is more cumbersome and complex, requiring more specialized technical personnel for maintenance and management. Moreover, due to the high power consumption of GPUs, their cooling requirements are also higher, necessitating additional cooling equipment and maintenance costs.
With the rapid development of AI technology, the performance of GPUs is continuously improving. To maintain competitiveness, many companies need to frequently purchase new versions of GPUs, which will increase the cost of servers.
As AI applications are promoted, more and more enterprises are beginning to use AI servers, leading to an increasing demand for GPUs. In the case of supply not meeting demand, the price of GPUs will also rise.Competitors are gearing up, with AMD being the most eye-catching, showing excellent performance recently.
On July 30th, AMD released its financial report for the second quarter of 2024, with a net profit surge of 881% year-on-year, and the data center business revenue doubled, taking away a lot of business from Nvidia.
AMD's total revenue for this quarter reached $5.835 billion, not only exceeding the expected $5.72 billion, but also achieving a year-on-year increase of 9% and a quarter-on-quarter increase of 7%. The net profit reached $265 million, with a year-on-year increase of 881% and a quarter-on-quarter increase of 115%.
The sales of the data center GPU chip MI300 exceeded $1 billion in a single quarter, driving a significant increase in the data center department's revenue.
The MI300 series is an AI GPU released by AMD at the end of 2023, including MI300X and MI300A, which integrates CPU cores and GPU accelerators. Among them, MI300X is positioned against Nvidia's H100. According to AMD, the performance of MI300X for AI training is on par with Nvidia's H100, and in terms of inference, its performance exceeds that of competitors. Taking a single server with 8 GPUs as an example, when running the 176 billion-parameter BLOOM model and the 70 billion-parameter Llama2 model, the MI300X platform's performance is 1.4 to 1.6 times that of the H100 platform.
AMD CEO Dr. Lisa Su stated that the company's AI chip sales were "better than expected", and Microsoft's usage of MI300 chips is increasing to support the computing power of GPT-4 Turbo and support Microsoft's Word, Teams, and other Copilot services. Hugging Face is one of the first customers to adopt the new Microsoft cloud Azure, enabling enterprises and AI customers to deploy hundreds of thousands of models on MI300 with a single click.
In June of this year, AMD announced its iterative roadmap, planning to launch MI325X in the fourth quarter of this year, and to successively launch the MI350 series and MI400 series in the next two years. Among them, M1300X and MI325X will use the CDNA3 architecture, M1350 will use the CDNA4 structure, and MI400 will use the next-generation CDNA architecture. AMD will launch a new product series every year thereafter. In the industry's view, this speed is in line with the plan released by Nvidia.
In addition, Dr. Lisa Su stated that the demand for AI inference will be greater than that for training. AI PCs are an important part of the PC category, and the PC market is a good opportunity for AMD's business revenue growth.This year, AMD has accelerated its AI layout through investment. In July, the company spent $665 million to acquire Silo AI, the largest artificial intelligence laboratory in Europe. The company provides end-to-end AI-driven solutions, and this acquisition is considered an important step for AMD to catch up with Nvidia.
Lisa Su said that in addition to acquiring Silo AI, AMD has invested more than $125 million in more than a dozen artificial intelligence companies over the past 12 months to expand the AMD ecosystem and maintain the leading position of AMD's computing platform. She said that AMD will continue to invest in software, which is one of the reasons for investing in Silo AI.
Based on high-quality GPU hardware, developing software and ecosystem, AMD is competing with Nvidia in the way of its success.
04
Nvidia also has weaknesses
To compete with Nvidia, it is the best policy to play to strengths and avoid weaknesses, that is, to leverage one's own advantages and attack Nvidia's shortcomings.
Although the parallel processing capability of GPU is very strong, which is also the fundamental reason for its proficiency in AI training. However, when data moves back and forth, the processing speed of the GPU is not fast. When running large AI models, a large number of GPUs and a large number of memory chips are often needed, which are interconnected. The faster the data moves between the GPU and memory, the better the performance. When training large AI models, some GPU cores will be idle, and almost half of the time is spent waiting for data.
If a large number of processor cores and a huge amount of memory can be combined to form in-memory computing, it can greatly reduce the connection complexity between multiple chips and greatly improve the data transfer speed. A large number of processor cores are connected inside the chip, and their running speed is hundreds of times faster than the independent GPU combination. At present, several start-ups are doing this, and the development situation is worth paying attention to.
In addition, it is necessary to be prepared for a protracted war in terms of software and hardware ecology in order to compete with Nvidia. In this regard, it is necessary to have a strong resource background to have the life to compete. AMD and Intel are doing this.
Furthermore, in addition to the chip itself, more effort can be made in the interconnection between chips. Nvidia is not the leader in this area, Broadcom is.Broadcom addresses the interconnectivity issues between chips, not directly competing with Nvidia's GPUs. Although Nvidia also has its own inter-chip interconnect technology, from an industry-wide perspective, Broadcom's technology and products are superior. Among the world's top 8 AI server systems, 7 have deployed Ethernet infrastructure supported by Broadcom's technology. It is expected that by 2025, all hyperscale AI server systems will be supported by Ethernet.
Broadcom excels at solving communication bandwidth issues. In the global market for 50GB/s SerDes, Broadcom holds a 76% share. Its SerDes interface converts low-speed parallel data into high-speed serial data, which is then converted back to parallel data at the receiving end. Through this operation, data can be transferred from one TPU to another at high speed, greatly improving transmission efficiency.
Similarly benefiting from the growth of AI, Broadcom's netcom product revenue is growing at an annual rate of 40%. The company's financial report shows that in the second fiscal quarter ending in May this year, AI revenue increased by 280% year-on-year, reaching $3.1 billion, and it is expected that this figure will exceed $11 billion by the end of the current fiscal year.
05
A big fall followed by a big rise
The pressure from a group of competitors is an important reason for the decline in Nvidia's stock price. However, the speed of market changes is too fast for people to react.
On the evening of July 31, Nvidia's stock price suddenly surged, with an increase of more than 14% at one point, adding $326.9 billion to its market value in a single day.
Nvidia has become the first stock to increase its market value by more than $300 billion in a single day. Currently, in the US stock market, Nvidia occupies the top three positions in terms of daily market value increase. On February 22 and May 23 this year, Nvidia's market value increased by $276.6 billion and $217.7 billion, respectively.
Morgan Stanley published a research report pointing out that considering Nvidia has been recently sold off in the market, although the specific reasons are unknown, it is believed to provide a good entry opportunity for interested investors. Therefore, it has been reclassified as the preferred stock, with unchanged profit forecasts and target price, rated as "buy", with a target price of $144.
In just two days, Nvidia's stock price fell sharply and then rose sharply, which may be related to the tight supply of Blackwell, making it difficult to deliver all on time.Morgan Stanley stated that the Blackwell product has sparked strong interest in the market, especially its significant improvement in inference performance, which further drives customers' desire to purchase. However, industry news has emerged that the Blackwell GPU chip may be delayed, or the server products equipped with this chip may be delayed.
Although the technology and products of many competitors are getting better and better, bringing pressure to Nvidia, the company's GPU products are still the main force in the AI server market in the current and near future, with overall supply not meeting demand. As the eagerly anticipated Blackwell GPU is about to be shipped, the news of delivery delays will surely pique the market's appetite, which is conducive to the rise in stock prices.
Comments